Black Swan - Data portability/ mobility and data sharing economy
I saw 50 black swans when thinking how data data portability/ mobility will migrate value towards the individual. Now some headlines are set up for click bait; however here is my picture taken in on the North Island New Zealand on lake Rotomahana when out walking and preparing this. Yes each little do it a black swan.
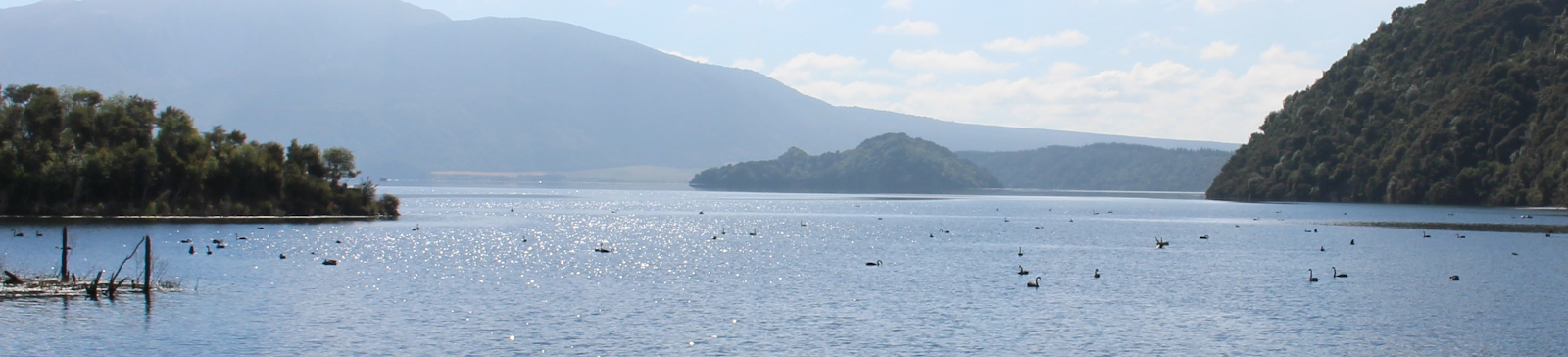
Summary: Been thinking about the complex and hidden implications of the personal data portability/ mobility models and data sharing economics. The thinking leads to the possibility of making it far harder for large silo data owners to sell/ share their data due to risk of re-identification; which changes the data economy. Less general silo data being available for sale but increasing demand for ‘quality’ data could mean individual collated data becomes far more valued far quicker than forecast, as the value chain shifts in response to new legislation/ regulation. Early and fast adopting countries will benefit with significant increases in innovation, investment and inward investment.
The start of this was a directed ideation from Ian Oppermann NSW Chief Data Scientist and CEO of NSW Data Analytics Centre. The Challenge: How do you design a privacy preserving data sharing framework based on these papers. They are well written and provide a very good framework for the question
I teamed up with Nathan Brewer, we drank coffee and came up with this flow diagram. He is the main man behind understanding the real depth of this and not the superficial layer this presents.

Purpose: I would like to share Data-Set D1 (and you have consent to share the data), so now is it safe to do so, and will we be able to preserve (individual) privacy
Assumption. Preserving Privacy in this case means that the individual cannot be (re)-identified.
Assumption. As an individual I have invoked my rights and collected (and will continue to collect/ stream) all my data into one secure place where I have a copy of all my data. (Dall) This is part of data portability/ mobility, the other part being I have asked someone else to collect / stream my silo'ed data, on my behalf, into their secure place.
Assumption. Personal Identifiable (PI) data is uniquely attachable and identifies an individual. Unique data is not personal but only 1. Looking for delta in risk from combined data sets where two or more unique enable identification when data sets are combined.
Assumption. You don't need any process if you have removed ALL [PI & unique data] from D1 and sufficiently desensitised data in D1 to the point that no-one individual could ever be re-identified using any other data set. However this assumption translates into D1 will have a low value and use. As an example, let’s take taxi data as D1 . If we have the pick up and drop of times to milliseconds and location to lat/long. Let’s desensitise this to hour and postcode. Using phone records as my second data set; I can easily get back to individuals (it may take a while but it is very possible). If the D1 data is desensitised to AM/ PM and county - it is still possible that a few individual who take long journeys across a boundary lines may be re-identified. (harder but possible) To be sure, if I want to share D1 in public, I would have to desensitised to probably date and country. At that level the D1 data set has some value for town planners but would not be sufficient for congestion, hence the value of any datasets economics change with both the level of sensitivity of the data and the volume of data (obvious).
----
The flow. Starting top right and working the way left
Question 1. It there any unique identifiers or PI in the data set D1. The lookup table is ISO/IEC 27018:2019(en) - this can be supplemented with expert opinion as required. Over time we will learn and this will become a fully automated process. Today it needs people and process.
If Yes there is PI or unique data, then you may share the data, but it would have to be in a very safe way or to a safe, trusted and contracted party and with clear controls. In this case, sharing realistically can only be another internal business unit/ department. Data must not leave the organisation, however in many businesses this itself can be an unsafe practice but that decision is controlled by their governance and processes. If the other party is untrusted you cannot share the data.
Question 2. Do I want to want to share/ release D1 with a specific external party or with the general public? The difference between these two scenarios is about the assumption to be made as to what data is available through the third party - that when combined with D1 creates re-identification probability. If the desire is to share with a known party, the request is to use their dataset to determine if when D1 and Dknown are combined, if and how many individuals can be identified?
If releasing D1 to the public, you must assume that a copy of all data (Dexternal ) is available as this is the worst case and now you need to test if an individual in D1 can be re-identified from the combined data in Dexternal . In this case let’s assume that Dexternal = Dall (this is all unique data set for an individual for all their data that they have gathered together in one place) Will include but not limited to social (public and private), health, wellness, financial, utilities, government, professional, messaging, location, web browsing, shopping and media. It is somewhat easier to understand that as an individual if I consented for my data to be combined with any public dataset, would my data help in the re-identification of me; as when one is done the next one(s) become(s) easier and the risk goes up.
Uniqueness test/ generate a result. Here D1 is combined with Dknown /Dexternal (Dall ) to create a result to indicate if D1 can be shared and preserve privacy. The critical part here is not the actual test or the maths/ analysis or stats but the acceptable mask/ level of risk that the test generates, as passing the mask will enable you to realise data, failure (outside of mask limits) will mean don’t share the data. What this overlay mask looks like as a standard is not determined as yet - but let’s assume it does and it is something we should work towards. In the example below - D1 is run with various desensitisation settings on the columns of data, the black black line shows the results, the dashed gray line shows the mask level that the data has to be below for public release.
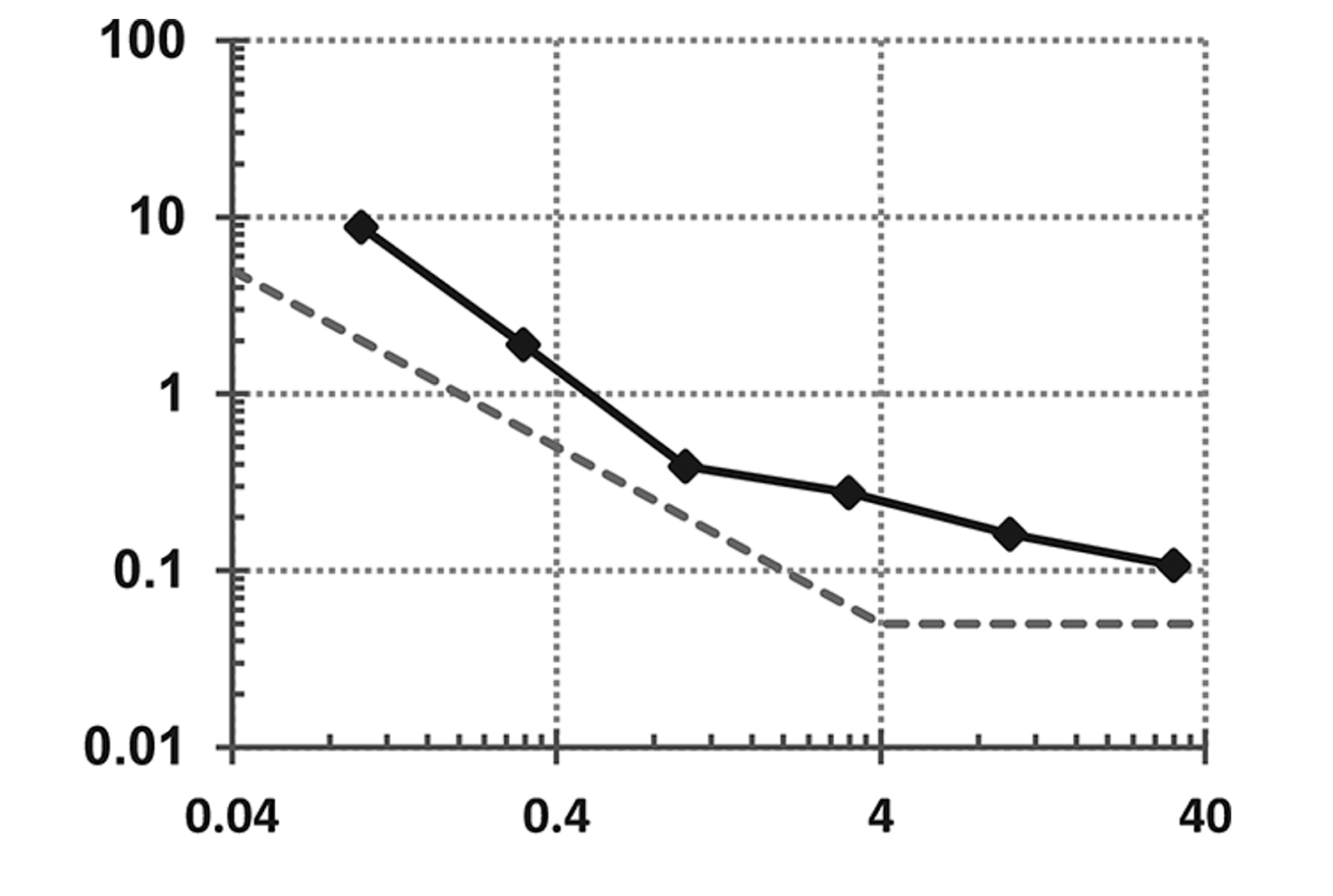
Observations
When sharing a data-set (D1 ) in public every column that has a unique identifier has to be desensitised, to a margin (which needs to be defined ) that means that when two data sets are in union, that no-one person can be uniquely identified. Learning: just desensitising D1 is a waste of time without the context of external data, and due to data portability/ mobility the external data source should be assumed to be all data about an individual (Dall ) If this is the case (Dall ) has the probability of being availability, then sharing any dataset becomes a massive challenge.
A question with the mask is becomes, does risk become the measurable possibility of one person in a data set being identified, or 10 people, or 100 people …. Or everyone. The mask line represents the risk in relation to how many individuals will be identified.
So whilst we must maintain the right of citizens to move/ copy/ share their data, should we also think about preventing anyone/body having full access to all personal data once it is in one place and also prevent the right to copy all data ( yes I too get the wider issue; so maybe to only pre-approved intermediaries) so as to protect the user from abuse and to enable more data sharing and value to be maintained in the existing models. , An comment is surly, would a regulator be able to understand and control this this level of consent?
---
There are a number of complex and hidden implications of the personal data portability/ mobility models when thinking about the data sharing economy (and wanting to offer privacy preserving sharing). If all data on individuals is available then useful silo data cannot be sold as the risk of re-identification becomes too high and by the time silo data is desensitised its value is lost.
However, since individual data is now available, do we shift the value chain from value creation of silo owners to value with the individuals and the platforms that support this new model? Those who sell access to individuals (not the data itself) e.g. Facebook’s model will also change as the consent will move to the individual and away from the general terms and conditions - interesting times for consent and governance.
Does that also translate into early and fast adopting countries will benefit with significant increases in innovation, investment and inward investment as entrepreneurs can benefit from favourable regulatory regimes and bring about a new data economy!
Summary: Been thinking about the complex and hidden implications of the personal data portability/ mobility models and data sharing economics. The thinking leads to the possibility of making it far harder for large silo data owners to sell/ share their data due to risk of re-identification; which changes the data economy. Less general silo data being available for sale but increasing demand for ‘quality’ data could mean individual collated data becomes far more valued far quicker than forecast, as the value chain shifts in response to new legislation/ regulation. Early and fast adopting countries will benefit with significant increases in innovation, investment and inward investment.
The start of this was a directed ideation from Ian Oppermann NSW Chief Data Scientist and CEO of NSW Data Analytics Centre. The Challenge: How do you design a privacy preserving data sharing framework based on these papers. They are well written and provide a very good framework for the question
- Privacy in Data Sharing – A Guide for Business and Government (Nov 2018)
- Data Sharing Frameworks – Technical White paper (Sept 2017)
I teamed up with Nathan Brewer, we drank coffee and came up with this flow diagram. He is the main man behind understanding the real depth of this and not the superficial layer this presents.
Purpose: I would like to share Data-Set D1 (and you have consent to share the data), so now is it safe to do so, and will we be able to preserve (individual) privacy
Assumption. Preserving Privacy in this case means that the individual cannot be (re)-identified.
Assumption. As an individual I have invoked my rights and collected (and will continue to collect/ stream) all my data into one secure place where I have a copy of all my data. (Dall) This is part of data portability/ mobility, the other part being I have asked someone else to collect / stream my silo'ed data, on my behalf, into their secure place.
Assumption. Personal Identifiable (PI) data is uniquely attachable and identifies an individual. Unique data is not personal but only 1. Looking for delta in risk from combined data sets where two or more unique enable identification when data sets are combined.
Assumption. You don't need any process if you have removed ALL [PI & unique data] from D1 and sufficiently desensitised data in D1 to the point that no-one individual could ever be re-identified using any other data set. However this assumption translates into D1 will have a low value and use. As an example, let’s take taxi data as D1 . If we have the pick up and drop of times to milliseconds and location to lat/long. Let’s desensitise this to hour and postcode. Using phone records as my second data set; I can easily get back to individuals (it may take a while but it is very possible). If the D1 data is desensitised to AM/ PM and county - it is still possible that a few individual who take long journeys across a boundary lines may be re-identified. (harder but possible) To be sure, if I want to share D1 in public, I would have to desensitised to probably date and country. At that level the D1 data set has some value for town planners but would not be sufficient for congestion, hence the value of any datasets economics change with both the level of sensitivity of the data and the volume of data (obvious).
----
The flow. Starting top right and working the way left
Question 1. It there any unique identifiers or PI in the data set D1. The lookup table is ISO/IEC 27018:2019(en) - this can be supplemented with expert opinion as required. Over time we will learn and this will become a fully automated process. Today it needs people and process.
If Yes there is PI or unique data, then you may share the data, but it would have to be in a very safe way or to a safe, trusted and contracted party and with clear controls. In this case, sharing realistically can only be another internal business unit/ department. Data must not leave the organisation, however in many businesses this itself can be an unsafe practice but that decision is controlled by their governance and processes. If the other party is untrusted you cannot share the data.
Question 2. Do I want to want to share/ release D1 with a specific external party or with the general public? The difference between these two scenarios is about the assumption to be made as to what data is available through the third party - that when combined with D1 creates re-identification probability. If the desire is to share with a known party, the request is to use their dataset to determine if when D1 and Dknown are combined, if and how many individuals can be identified?
If releasing D1 to the public, you must assume that a copy of all data (Dexternal ) is available as this is the worst case and now you need to test if an individual in D1 can be re-identified from the combined data in Dexternal . In this case let’s assume that Dexternal = Dall (this is all unique data set for an individual for all their data that they have gathered together in one place) Will include but not limited to social (public and private), health, wellness, financial, utilities, government, professional, messaging, location, web browsing, shopping and media. It is somewhat easier to understand that as an individual if I consented for my data to be combined with any public dataset, would my data help in the re-identification of me; as when one is done the next one(s) become(s) easier and the risk goes up.
Uniqueness test/ generate a result. Here D1 is combined with Dknown /Dexternal (Dall ) to create a result to indicate if D1 can be shared and preserve privacy. The critical part here is not the actual test or the maths/ analysis or stats but the acceptable mask/ level of risk that the test generates, as passing the mask will enable you to realise data, failure (outside of mask limits) will mean don’t share the data. What this overlay mask looks like as a standard is not determined as yet - but let’s assume it does and it is something we should work towards. In the example below - D1 is run with various desensitisation settings on the columns of data, the black black line shows the results, the dashed gray line shows the mask level that the data has to be below for public release.
Observations
When sharing a data-set (D1 ) in public every column that has a unique identifier has to be desensitised, to a margin (which needs to be defined ) that means that when two data sets are in union, that no-one person can be uniquely identified. Learning: just desensitising D1 is a waste of time without the context of external data, and due to data portability/ mobility the external data source should be assumed to be all data about an individual (Dall ) If this is the case (Dall ) has the probability of being availability, then sharing any dataset becomes a massive challenge.
A question with the mask is becomes, does risk become the measurable possibility of one person in a data set being identified, or 10 people, or 100 people …. Or everyone. The mask line represents the risk in relation to how many individuals will be identified.
So whilst we must maintain the right of citizens to move/ copy/ share their data, should we also think about preventing anyone/body having full access to all personal data once it is in one place and also prevent the right to copy all data ( yes I too get the wider issue; so maybe to only pre-approved intermediaries) so as to protect the user from abuse and to enable more data sharing and value to be maintained in the existing models. , An comment is surly, would a regulator be able to understand and control this this level of consent?
---
There are a number of complex and hidden implications of the personal data portability/ mobility models when thinking about the data sharing economy (and wanting to offer privacy preserving sharing). If all data on individuals is available then useful silo data cannot be sold as the risk of re-identification becomes too high and by the time silo data is desensitised its value is lost.
However, since individual data is now available, do we shift the value chain from value creation of silo owners to value with the individuals and the platforms that support this new model? Those who sell access to individuals (not the data itself) e.g. Facebook’s model will also change as the consent will move to the individual and away from the general terms and conditions - interesting times for consent and governance.
Does that also translate into early and fast adopting countries will benefit with significant increases in innovation, investment and inward investment as entrepreneurs can benefit from favourable regulatory regimes and bring about a new data economy!